The Internet of Things (IoT) is rapidly transforming our world, with interconnected devices monitoring everything from home thermostats to industrial machinery. But managing a vast network of these devices can be a challenge. Imagine a malfunctioning sensor disrupting your smart irrigation system, or a glitch in a connected medical device leading to critical delays. Here’s where AI and Machine Learning (ML) come to the rescue, enabling proactive monitoring of IoT networks for optimal performance.
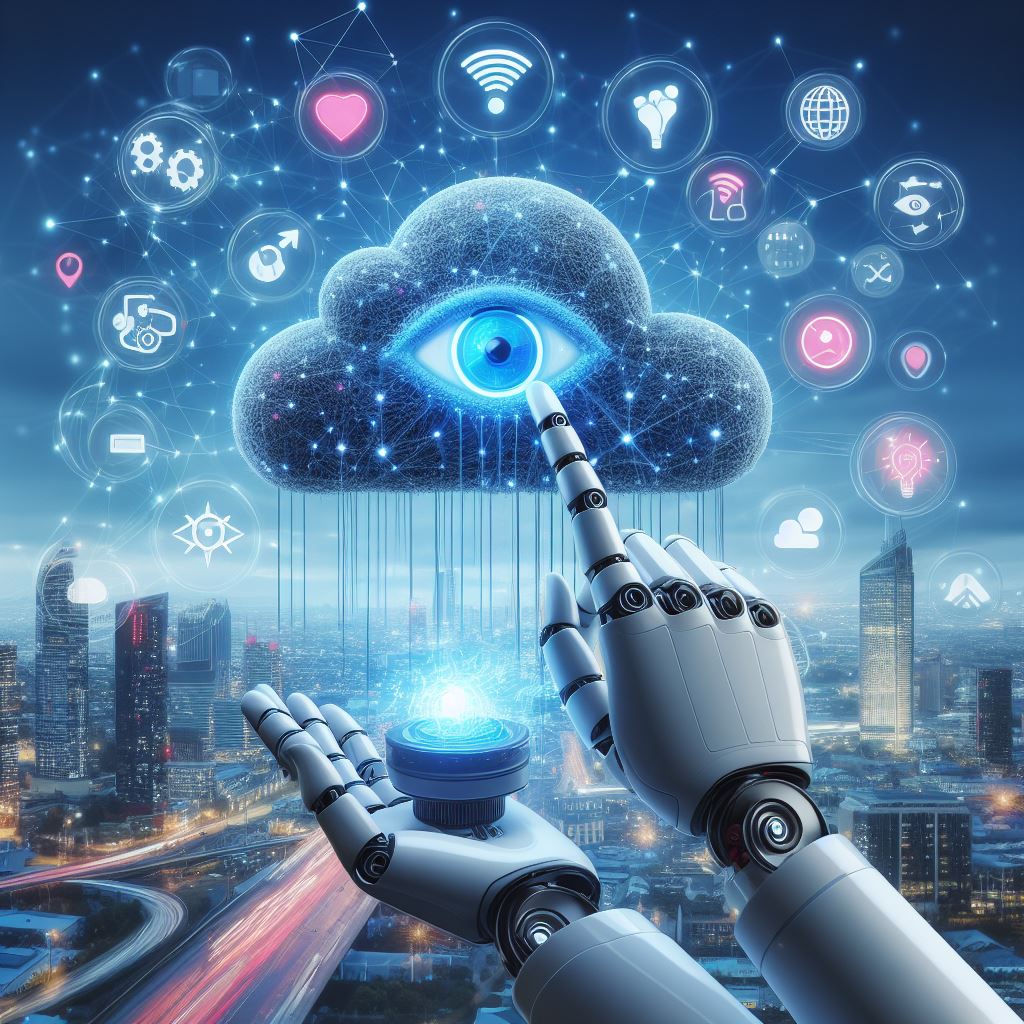
From Reactive to Predictive: Anomaly Detection for the IoT Age
Traditionally, identifying and resolving issues in IoT networks often relied on user complaints or reactive monitoring systems. AI/ML flips the script by enabling proactive anomaly detection. These intelligent algorithms analyze vast amounts of data – sensor readings, device status updates, and network traffic patterns – to establish a baseline for normal behavior within the IoT network.
Deviations from this baseline, such as a sudden spike in power consumption from a sensor or a drop in data transmission rates, are flagged as anomalies. By identifying these anomalies in real-time, AI/ML empowers technicians to address potential problems before they snowball into widespread outages or impact critical systems.
The Crystal Ball Effect: Predicting Device Disruptions
ML doesn’t just focus on the present; it can also predict the future, at least when it comes to IoT device performance. By analyzing historical data, ML models can identify trends and patterns that have preceded device failures or network disruptions in the past. This allows for preventative maintenance – scheduling software updates, battery replacements, or network optimizations before issues arise.
Think of it like predicting equipment failure in a factory based on vibration sensor readings. Proactive maintenance in the IoT world translates to fewer device malfunctions, smoother data flow, and a more reliable overall network.
Getting to the Root of the Glitch: AI-powered Root Cause Analysis
Despite proactive measures, issues can still occur within an IoT network. But what if troubleshooting wasn’t a time-consuming mystery? AI/ML can analyze network data during a disruption to pinpoint the exact cause of the problem. This could be anything from a faulty sensor to communication interference or even a cyberattack.
Faster root cause analysis translates to quicker fixes, minimizing downtime and ensuring critical IoT systems remain operational. Additionally, by identifying recurring patterns, AI/ML can help prevent similar issues from happening again, leading to a more robust and resilient IoT network.
The Future of IoT: Powered by AI/ML
The integration of AI/ML in IoT network monitoring is a significant leap forward. By enabling real-time anomaly detection, predictive maintenance, and intelligent root cause analysis, these technologies are paving the way for a future where disruptions in the Internet of Things are a rarity.
So, the next time your smart devices seamlessly connect and function as intended, remember, there might be some clever AI/ML working behind the scenes to keep the Internet of Things running smoothly.